Forecasting COVID-19 trends became challenging in the second half of 2021, because it is difficult to estimate effects of vaccination and waning immunity, especially without access to finely disaggregated surveillance data. As a result, regional modeling efforts had to use vaccine efficacy, vaccine effectiveness, and rates of immunity waning estimated outside of the population of interest. Inherent uncertainty of these parameters and potential lack of their portability to the population of interest resulted in highly variable forecasts and scenarios. We propose to develop Bayesian data integration that will use multiple streams of regional disaggregated surveillance data (cases, tests, hospitalization, deaths) to estimate vaccine effectiveness, rates of immunity waning, and changes in the transmission rate in real time. The proposed data integration, tailored for each geographic region of interest (e.g., county or city), should provide California policy makers with sharper forecasts and a narrower range of scenarios.
Other Modeling and Advanced Analytics Funded Projects
Investigating Interventions for Responding to Misinformation (IN²FORM)
Image
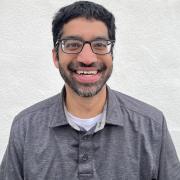
Ravi Goyal
UCSD
Image
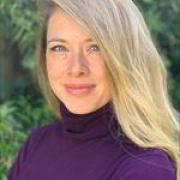
Natasha K. Martin
UCSD
Active